An Artificial Intelligence Based Multi-Epitope Vaccine for COVID-19 (SARS-CoV-2 Virus)
Dr. Amit Ray
Compassionate AI Lab
Effective and early vaccine design is the key challenge in the present COVID crisis. We reviewed, DeepVaxAI, an Artificial Intelligence based multi-epitope vaccine development system, one of our key project at compassionate AI Lab to fight against COVID virus. The objective is to develop most reliable peptide based in-silico vaccines for any given protein sequences with minimum turn-around time. The key objective of our Compassionate AI lab is to eliminate pain of the humanity. This project is part of that endeavor.
Presently humanity is going through tremendous loss and suffering due to coronavirus pandemic. Humanity is desperately looking for an urgent solution to come out of this urgent situation. Developing safe and effective vaccine is one of the key solutions for the present crisis. However, long turn-around time for vaccine development is the key obstacle for effective fight against COVD-19 pandemic. In this article, we reviewed DeepVaxAI; the Artificial Intelligence based multi-epitope vaccine development workflow process automaton system. The system architecture of the AI based automatic peptide based vaccine design is shown in the figure below.
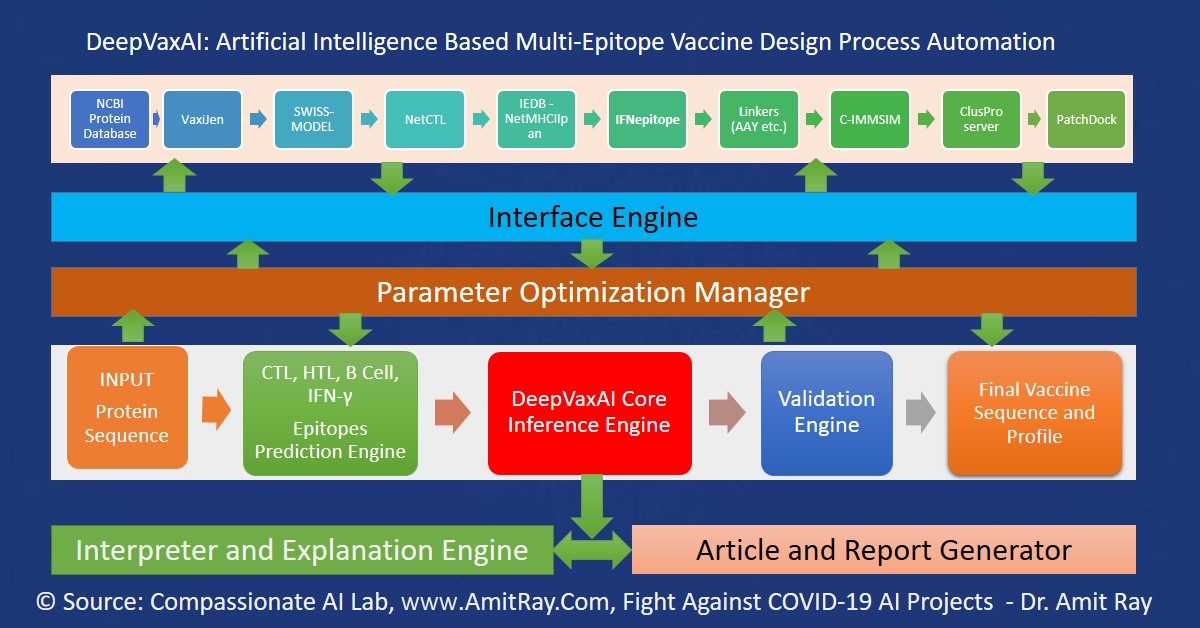
AI Based Vaccine Design System Architecture
The key objective of vaccination is to stimulate the immune system, the natural disease-fighting capabilities of the body. To develop peptic based vaccine epitopes the following properties are usually preferred: highly antigenic, highly non-allergic, highly non-toxic, significant population coverage, having a strong binding affinity with common human allele.
System Architecture of the AI Based Vaccine Design System
The interface engine, part of the DeepVaxAI system provides extensive links to major internal and external databases. Moreover, the interface engine reduces the workload of the research scientists. The key servers linked to the interface engine are NCBI database, IEDB server, NetCTL, VaxiJen, Toxinpred server, ERRAT server, GRAMM-X Simulation web server, LIGPLUS server, AllerTOP, ProtParam, C-ImmSim server, SwissDock, PatchDock, HADDOCK, and YASARA server.
Traditional methods of peptide based vaccine development process is time-consuming, monotonous and very labor-intensive. However, Deep Artificial intelligence (Deep AI) is a key technology for optimizing the process flow in many application areas. Here, we especially focus for the vaccine development. Our COVID-19 vaccine design protocol is very effective , easy and systematic.
This vaccine design protocol can improve process accuracy and remove potential human biases, errors, and repetitions. Moreover, it will substantially reduce the vaccine development turn-around times. Further, the protocol, will enable subject matter experts to focus more on higher value tasks like wet lab experiments. The gap between wet lab experiments and in-silico studies is the key obstacle for vaccine development. With the help of DeepVaxAI, research scientists can now focus more on wet lab experiments and eliminate the gap.
The core inference engine part of the DeepVaxAI system provides facilities for modeling with various AI algorithms. Traditionally, shallow AI includes narrow areas and build models with deep learning algorithms. Deep learning algorithms like MLP, DNN, CNN, RNN, LSTM are powerful. However, they have many limitations.
On the other hand, Deep AI includes integration and collaboration of many technologies to provide the highest level of machine intelligence, process automaton, automatic interpretation, explanation, report generation, and scientific article generation capabilities. Research scientists will get more time for wet lab experiments, where the true solution exists.
15 Key Steps for Multi Epitope Vaccine Design
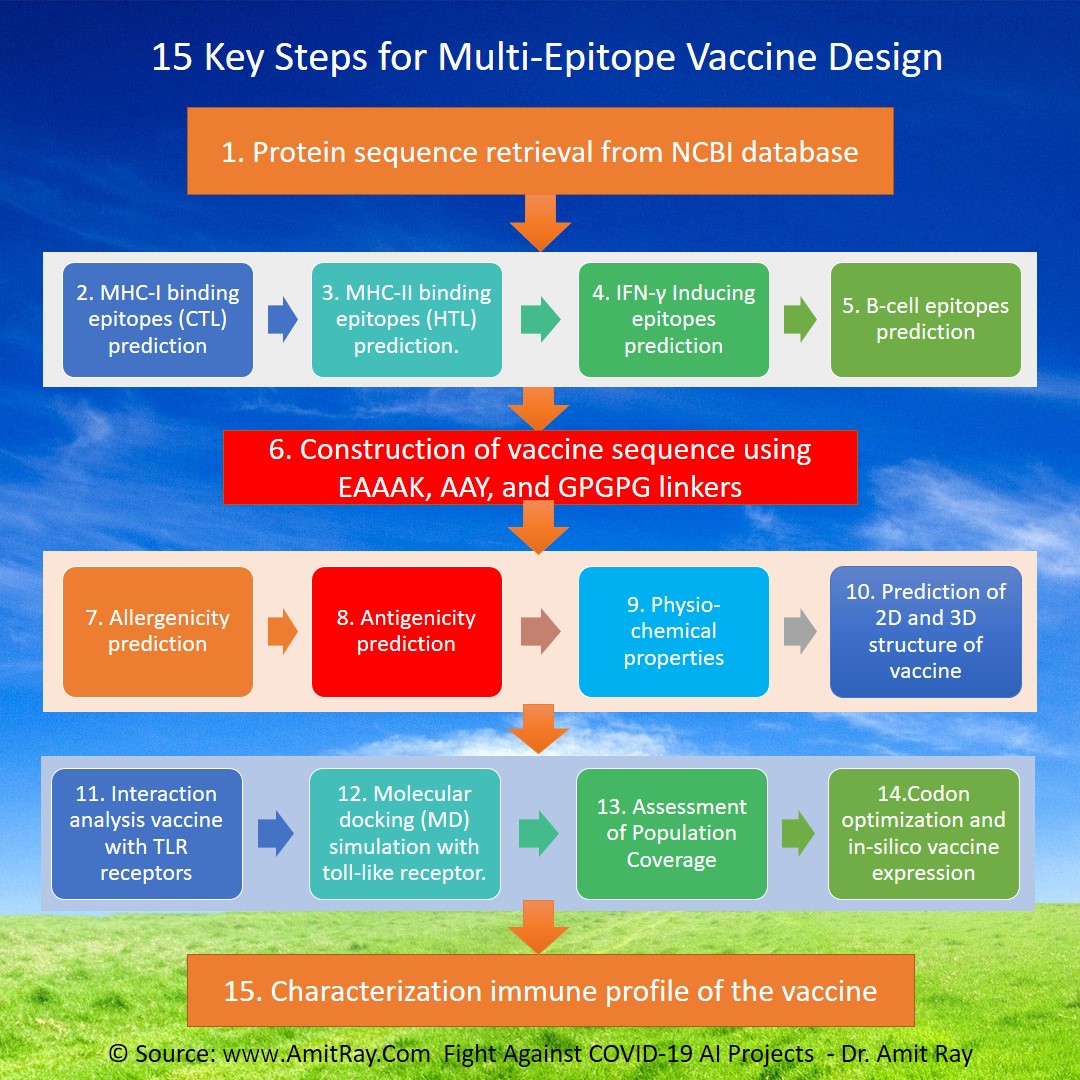
15 Key Steps for Multi Epitope Vaccine Design
The 15 primary steps for multi-epitope vaccine design are as follows:
- Retrieval of protein sequence from NCBI database
- MHC-I binding epitopes (CTL) prediction
- MHC-II binding epitopes (HTL) prediction.
- Prediction of IFN-γ Inducing Epitopes
- B-cell epitopes prediction
- Construction of vaccine sequence
- Calculating allergenicity of vaccine sequence
- Calculating antigenicity of vaccine sequence
- Reviewing physio-chemical properties of vaccine sequence
- Prediction of secondary and tertiary structure of vaccine
- Interaction analysis vaccine with TLR receptors
- Molecular docking (MD) simulation with toll-like receptor.
- Assessment of Population Coverage
- Codon optimization and in-silico vaccine expression
- Characterization of immune profile of the vaccine.
The 26 Top Vaccine Design Tools and Servers
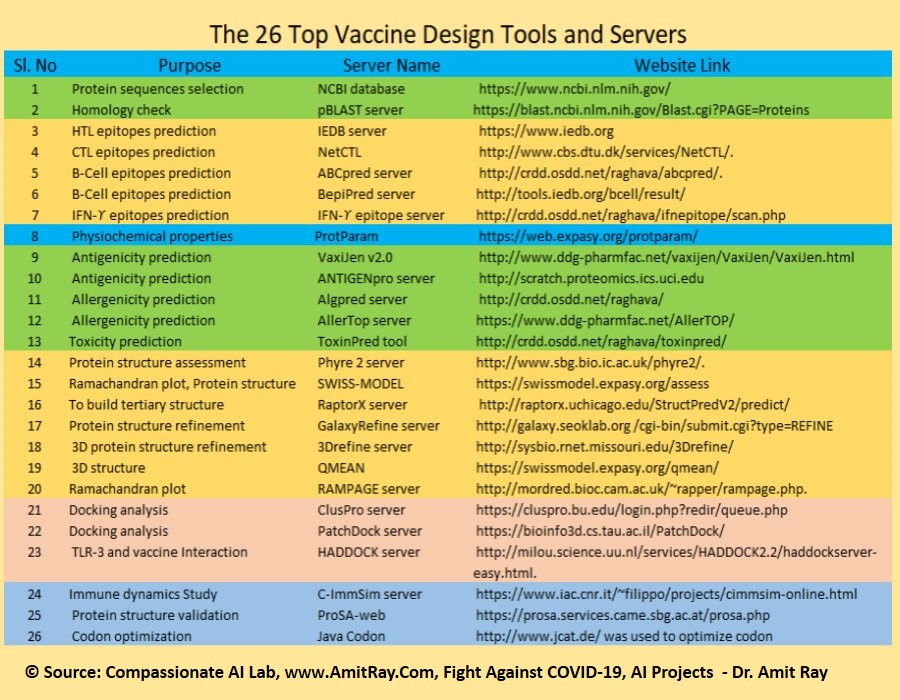
26 Top COVlD Vaccine Design Tools and Servers
Genomic Structure of SARS-CoV-2
The genome of SARS-CoV-2 is a single-stranded positive-sense RNA with the size of 29.8–30 kb encoding about 9860 amino acids. Moreover, the SARS-CoV-2 protein sequence includes 16 non-structural proteins (nsp1,nsp2, nsp3, .., nsp16), 4 structural proteins, (E, M, N and S) proteins, and accessory proteins (ORF3a, ORF7a, and ORF8).
The S, N, M, E form the structural proteins that play a vital role in the life cycle of the viral particles. The S protein is shaped like a clove with two subunits S1 and S2 which promotes receptor binding and membrane fusion respectively. The N protein enhances viral entry and performs post-fusion cellular processes necessary for viral survival and growth in the host. The E protein promotes virion formation and viral pathogenicity while M protein forms ribonucleoproteins and mediates inflammatory responses in hosts. Proteins ORF1a and ORF1ab are papain-like proteases (PL(pro)) involved in viral infection and are potential targets for the development of antiviral drugs.
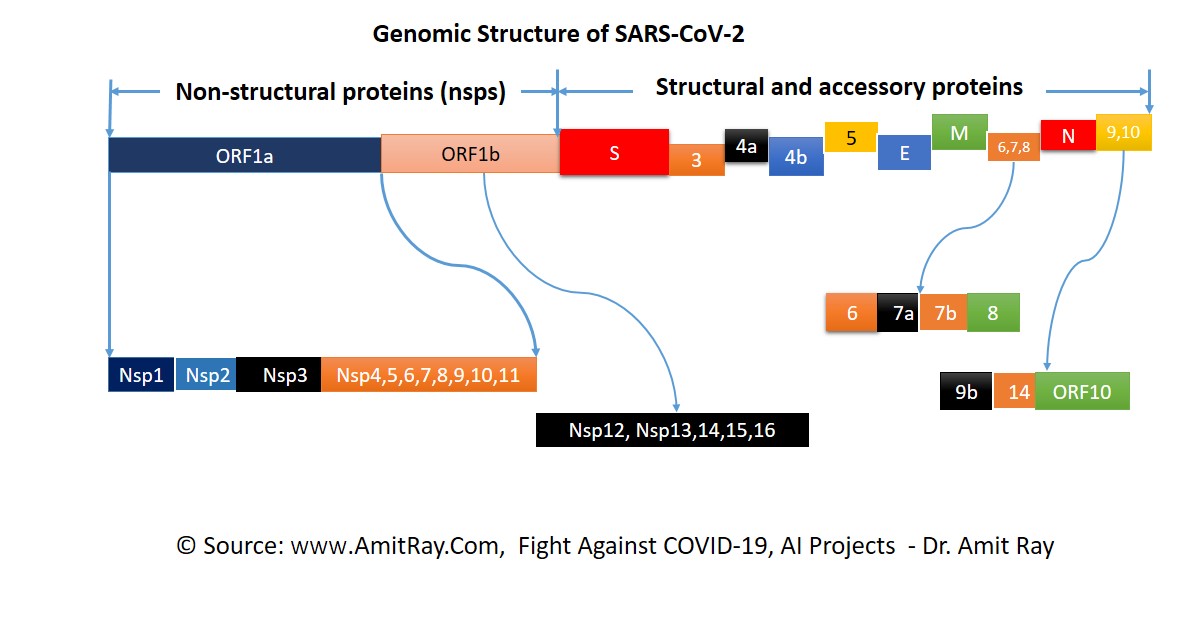
Genomic Structure of SARS-CoV-2
Unusually among coronaviruses, the SARS-CoV-2 S protein is proteolytically cleaved into an S1 subunit (685 amino acids) and an S2 membrane-spanning subunit (588 amino acids), the latter being highly conserved (99%) among CoV families. By contrast, S1 shows only 70% identity to other human CoV strains and the differences are concentrated in the RBD, which facilitates virus entry by binding to angiotensin-converting enzyme 2 (ACE2) on the host cell surface.
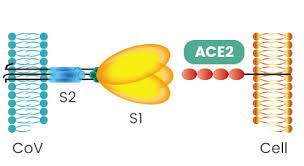
S Protein and ACE2 Binding
The Candidate Vaccine against SARS-CoV-2
In this paragraph, we will explain the key candidates for COVID-19 vaccines. One of our objective is to reduce the turn-around time of the vaccine development process. Hence, we divided the entire study into several phases. Firstly, we have created 15 batches of protein sequences of COVID viruses, randomly selected from the NCBI database.
Our approach is to train the DeepVaxAI system by observing the human behavior of the workflow and parameter optimization, to better automate the end-to-end vaccine design processes. The main workflow includes epitope predictions (HTL, CTL, IFN-γ and B cell epitopes) from the chosen protein sequences; vaccine construction and its quality check. Molecular Docking with immune cell receptor, followed by molecular dynamics simulation (MDS) to check vaccine’s stability. In addition, codon adaptation and immune simulation are used to understand how the vaccine acquires an immune response.
Vaccine Linkers and Adjuvants
We analyzed various competitive candidate vaccines to fight against COVID viruses. Finally, we selected the vaccine construct consisted of 563 amino acid residues derived from different peptide sequences. The immunogenic epitopes were united with the help of linkers; B-cell (KK linkers), CTL (AAY linkers), HTL (GPGPG linkers), and IFN-γ (GPGPG linkers). To enhance vaccine immunogenicity adjuvant was added to the N-terminal of the vaccine with the aid of the EAAAK linker. We analyzed human β-defensin-2 (hBD-2), human β-defensin-3 (hBD-3) and Matrix-M1 as adjuvants to enhance the immunogenic response.
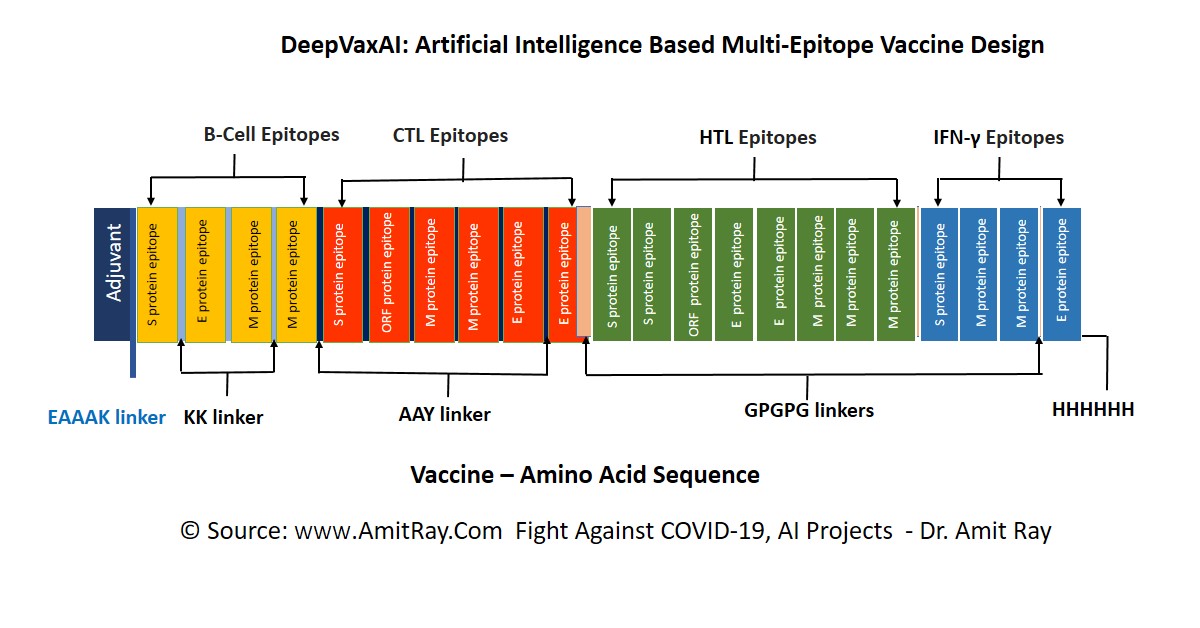
SARS-CoV-2 (COVID) Vaccine Amino Acid Sequence
Conclusion
In conclusion, we disused the system architecture, tools and techniques of the Deep-AI based vaccine design system. The system constructed a 563 amino acid based vaccine for COVID viruses. But there are still many obstacles to overcome. Manual interventions and checks are required in many places. Special care must be taken for final vaccine selection. For example, spike protein-based SARS vaccine often induce harmful immune responses that cause liver damages. We want the system to take care every possibility to provide the highest level of human safety.
Application of DeepVaxAI in silico methods can be used to design an effective vaccine in lesser time and low cost. Research scientists, process analysts, technical experts, and knowledge workers can drive unprecedented scale of automation while improving performance, accuracy, and data security. Deep-AI based vaccine design system is a powerful end-to-end automaton process harnessing the power of multiple technologies to improve accuracy, effectiveness, and to reduce time and cost for effective vaccine development.
Download: Artificial Intelligence Based COVID-19 Vaccine Design: A Guidebook By Dr. Amit Ray
The key tools used for vaccine design are as follows:
Sl. No | Purpose | Server Name | Website Link |
1 | Protein sequences selection | NCBI database | https://www.ncbi.nlm.nih.gov/ |
2 | Homology check | pBLAST server | https://blast.ncbi.nlm.nih.gov/Blast.cgi?PAGE=Proteins |
3 | HTL epitopes prediction | IEDB server | https://www.iedb.org |
4 | CTL epitopes prediction | NetCTL | http://www.cbs.dtu.dk/services/NetCTL/ |
5 | B-Cell epitopes prediction | ABCpred server | http://crdd.osdd.net/raghava/abcpred/ |
6 | B-Cell epitopes prediction | BepiPred server | http://tools.iedb.org/bcell/result/ |
7 | IFN-γ epitopes prediction | IFN-γ epitope server | http://crdd.osdd.net/raghava/ifnepitope/scan.php |
8 | Physiochemical property analysis | ProtParam | https://web.expasy.org/protparam/ |
9 | Antigenicity prediction | VaxiJen v2.0 | http://www.ddg-pharmfac.net/vaxijen/VaxiJen/VaxiJen.html |
10 | Antigenicity prediction | ANTIGENpro server | http://scratch.proteomics.ics.uci.edu |
11 | Allergenicity prediction | Algpred server | http://crdd.osdd.net/raghava/ |
12 | Allergenicity prediction | AllerTop server | https://www.ddg-pharmfac.net/AllerTOP/ |
13 | Toxicity prediction | ToxinPred tool | http://crdd.osdd.net/raghava/toxinpred/ |
14 | Protein structure assessment | Phyre 2 server | http://www.sbg.bio.ic.ac.uk/phyre2/ |
15 | Ramachandran plot & Protein structure | SWISS-MODEL | https://swissmodel.expasy.org/assess |
16 | Tertiary structure | RaptorX server | http://raptorx.uchicago.edu/StructPredV2/predict/ |
17 | Protein structure refinement | GalaxyRefine server | http://galaxy.seoklab.org /cgi-bin/submit.cgi?type=REFINE |
18 | 3D protein structure refinement | 3Drefine server | http://sysbio.rnet.missouri.edu/3Drefine/ |
19 | 3D structure | QMEAN | https://swissmodel.expasy.org/qmean/ |
20 | Ramachandran plot | RAMPAGE server | http://mordred.bioc.cam.ac.uk/~rapper/rampage.php |
21 | Docking analysis | ClusPro server | https://cluspro.bu.edu/login.php?redir/queue.php |
22 | Docking analysis | PatchDock server | https://bioinfo3d.cs.tau.ac.il/PatchDock/ |
23 | TLR-3 and vaccine Interaction | HADDOCK server | http://milou.science.uu.nl/services/HADDOCK2.2/haddockserver-easy.html. |
24 | Immune dynamics Study | C-ImmSim server | https://www.iac.cnr.it/~filippo/projects/cimmsim-online.html |
25 | Protein structure validation | ProSA-web | https://prosa.services.came.sbg.ac.at/prosa.php |
26 | Codon optimization | Java Codon | http://www.jcat.de/ |